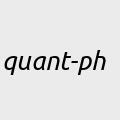
2024-04-08 08:45:35
This https://arxiv.org/abs/2307.15841 has been replaced.
initial toot: https://mastoxiv.page/@arXiv_qu…
This https://arxiv.org/abs/2307.15841 has been replaced.
initial toot: https://mastoxiv.page/@arXiv_qu…
"He is mandated to compile and share the achievements of the Islamic Revolution in culture, science, and art on his internet platform. Furthermore, he is required to prepare a summary of two books regarding women’s status in Islam, produce a song addressing “USA’s atrocities against humanity,” collect cases of human rights violations by U.S. governments over the past century, and participate in behavior and knowledge skills training courses in the field of art."
This https://arxiv.org/abs/2312.08864 has been replaced.
initial toot: https://mastoxiv.page/@arXiv_ees…
VeriEQL: Bounded Equivalence Verification for Complex SQL Queries with Integrity Constraints
Yang He, Pinhan Zhao, Xinyu Wang, Yuepeng Wang
https://arxiv.org/abs/2403.03193
Whoop I got accepted to #pifcamp ! Looking forward to it including working with @… and @… on some e-textile sensor sound stuff
"By 1973, the first volume of the defining work of our craft, “The Art of Computer Programming” had been available in bookstores since 1968, and its first chapter literally consisted of a 100-something page long introduction to various mathematical concepts. Induction, logarithms, series, matrices, elementary number theory, permutations and factorials, Fibonacci numbers, are some of the subjects exposed in those beautifully typeset pages."
For those of you who will still be there at #DHd2024 on Friday morning: if you want to know how to implement a #knowledgegraph based research data ecosystem (not only) for @nfdi4culture, please come and listen to our presentation on "Communities, Harvesting, and CGIF: Building the Research…
Learning general Gaussian mixtures with efficient score matching
Sitan Chen, Vasilis Kontonis, Kulin Shah
https://arxiv.org/abs/2404.18893 https://arxiv.org/pdf/2404.18893
arXiv:2404.18893v1 Announce Type: new
Abstract: We study the problem of learning mixtures of $k$ Gaussians in $d$ dimensions. We make no separation assumptions on the underlying mixture components: we only require that the covariance matrices have bounded condition number and that the means and covariances lie in a ball of bounded radius. We give an algorithm that draws $d^{\mathrm{poly}(k/\varepsilon)}$ samples from the target mixture, runs in sample-polynomial time, and constructs a sampler whose output distribution is $\varepsilon$-far from the unknown mixture in total variation. Prior works for this problem either (i) required exponential runtime in the dimension $d$, (ii) placed strong assumptions on the instance (e.g., spherical covariances or clusterability), or (iii) had doubly exponential dependence on the number of components $k$.
Our approach departs from commonly used techniques for this problem like the method of moments. Instead, we leverage a recently developed reduction, based on diffusion models, from distribution learning to a supervised learning task called score matching. We give an algorithm for the latter by proving a structural result showing that the score function of a Gaussian mixture can be approximated by a piecewise-polynomial function, and there is an efficient algorithm for finding it. To our knowledge, this is the first example of diffusion models achieving a state-of-the-art theoretical guarantee for an unsupervised learning task.
Integrating A.I. in Higher Education: Protocol for a Pilot Study with 'SAMCares: An Adaptive Learning Hub'
Syed Hasib Akhter Faruqui, Nazia Tasnim, Iftekhar Ibne Basith, Suleiman Obeidat, Faruk Yildiz
https://arxiv.org/abs/2405.00330
This https://arxiv.org/abs/2402.13178 has been replaced.
initial toot: https://mastoxiv.page/@arXiv_csCL_…
A Flow-based Credibility Metric for Safety-critical Pedestrian Detection
Maria Lyssenko, Christoph Gladisch, Christian Heinzemann, Matthias Woehrle, Rudolph Triebel
https://arxiv.org/abs/2402.07642
Integrating A.I. in Higher Education: Protocol for a Pilot Study with 'SAMCares: An Adaptive Learning Hub'
Syed Hasib Akhter Faruqui, Nazia Tasnim, Iftekhar Ibne Basith, Suleiman Obeidat, Faruk Yildiz
https://arxiv.org/abs/2405.00330
This https://arxiv.org/abs/2312.08976 has been replaced.
initial toot: https://mastoxiv.page/@arXiv_csSE_…
NeuralDiffuser: Controllable fMRI Reconstruction with Primary Visual Feature Guided Diffusion
Haoyu Li, Hao Wu, Badong Chen
https://arxiv.org/abs/2402.13809 https://arxiv.org/pdf/2402.13809
arXiv:2402.13809v1 Announce Type: new
Abstract: Reconstructing visual stimuli from functional Magnetic Resonance Imaging (fMRI) based on Latent Diffusion Models (LDM) provides a fine-grained retrieval of the brain. A challenge persists in reconstructing a cohesive alignment of details (such as structure, background, texture, color, etc.). Moreover, LDMs would generate different image results even under the same conditions. For these, we first uncover the neuroscientific perspective of LDM-based methods that is top-down creation based on pre-trained knowledge from massive images but lack of detail-driven bottom-up perception resulting in unfaithful details. We propose NeuralDiffuser which introduces primary visual feature guidance to provide detail cues in the form of gradients, extending the bottom-up process for LDM-based methods to achieve faithful semantics and details. We also developed a novel guidance strategy to ensure the consistency of repeated reconstructions rather than a variety of results. We obtain the state-of-the-art performance of NeuralDiffuser on the Natural Senses Dataset (NSD), which offers more faithful details and consistent results.
HDReason: Algorithm-Hardware Codesign for Hyperdimensional Knowledge Graph Reasoning
Hanning Chen, Yang Ni, Ali Zakeri, Zhuowen Zou, Sanggeon Yun, Fei Wen, Behnam Khaleghi, Narayan Srinivasa, Hugo Latapie, Mohsen Imani
https://arxiv.org/abs/2403.05763
CKERC : Joint Large Language Models with Commonsense Knowledge for Emotion Recognition in Conversation
Yumeng Fu
https://arxiv.org/abs/2403.07260 https://<…
Zero-Shot Code Representation Learning via Prompt Tuning
Nan Cui, Xiaodong Gu, Beijun Shen
https://arxiv.org/abs/2404.08947 https://a…
This https://arxiv.org/abs/2403.03193 has been replaced.
initial toot: https://mastoxiv.page/@arXiv_csPL_…
NeuralDiffuser: Controllable fMRI Reconstruction with Primary Visual Feature Guided Diffusion
Haoyu Li, Hao Wu, Badong Chen
https://arxiv.org/abs/2402.13809 https://arxiv.org/pdf/2402.13809
arXiv:2402.13809v1 Announce Type: new
Abstract: Reconstructing visual stimuli from functional Magnetic Resonance Imaging (fMRI) based on Latent Diffusion Models (LDM) provides a fine-grained retrieval of the brain. A challenge persists in reconstructing a cohesive alignment of details (such as structure, background, texture, color, etc.). Moreover, LDMs would generate different image results even under the same conditions. For these, we first uncover the neuroscientific perspective of LDM-based methods that is top-down creation based on pre-trained knowledge from massive images but lack of detail-driven bottom-up perception resulting in unfaithful details. We propose NeuralDiffuser which introduces primary visual feature guidance to provide detail cues in the form of gradients, extending the bottom-up process for LDM-based methods to achieve faithful semantics and details. We also developed a novel guidance strategy to ensure the consistency of repeated reconstructions rather than a variety of results. We obtain the state-of-the-art performance of NeuralDiffuser on the Natural Senses Dataset (NSD), which offers more faithful details and consistent results.
CKERC : Joint Large Language Models with Commonsense Knowledge for Emotion Recognition in Conversation
Yumeng Fu
https://arxiv.org/abs/2403.07260 https://<…
This https://arxiv.org/abs/2401.04079 has been replaced.
initial toot: https://mastoxiv.page/@arXiv_ees…
This https://arxiv.org/abs/2402.03216 has been replaced.
link: https://scholar.google.com/scholar?q=a